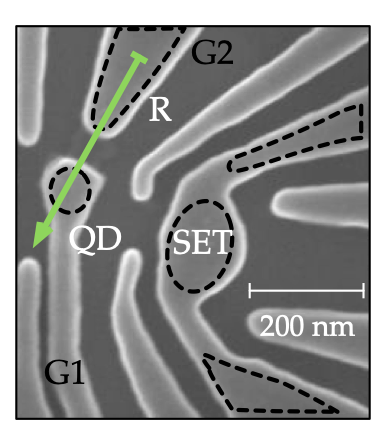
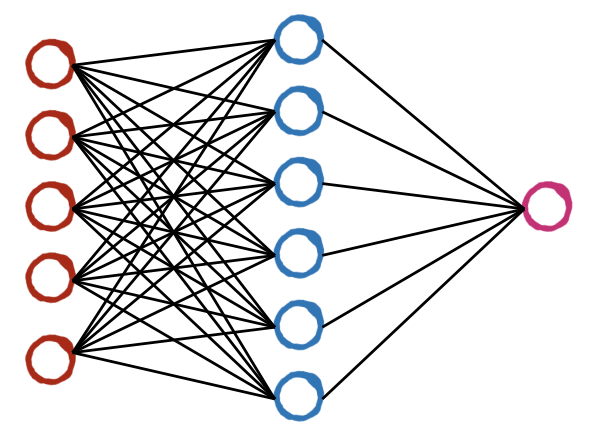
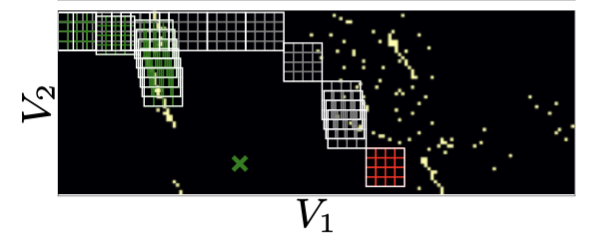
We use artificial neural networks to advance the setup of quantum experiments. This can be done by automatizing calibration and tuning tasks.
A common example are solid-state quantum dots which are realized in semiconducting devices. These devices require complicated and precise tuning processes at various stages. We use artificial neural networks to automatize these processes and reduce the human interaction with the experiment.
We additionally consider implementing vector-matrix multiplications as basic operations in artificial neural networks on accelerating analog hardware. This promises an efficient evaluation of the neural networks on the one hand, and an experimental integration close to the qubit system on the other hand.
Related publications
- S. Czischek, M. Pioro-Ladrière, D. Drouin, R. G. Melko, et al. Miniaturizing neural networks for charge state autotuning in quantum dots Mach. Learn.: Sci. Technol. 3, 015001 (2021)