Representing quantum states with artificial neural networks
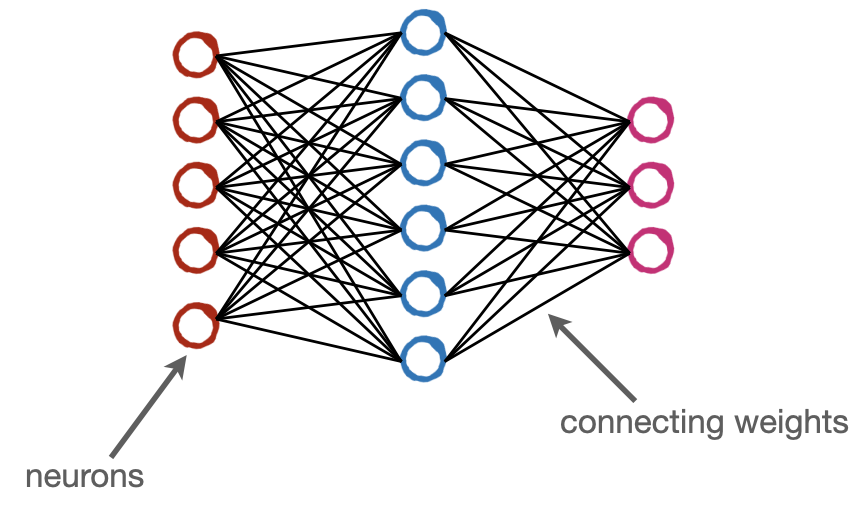
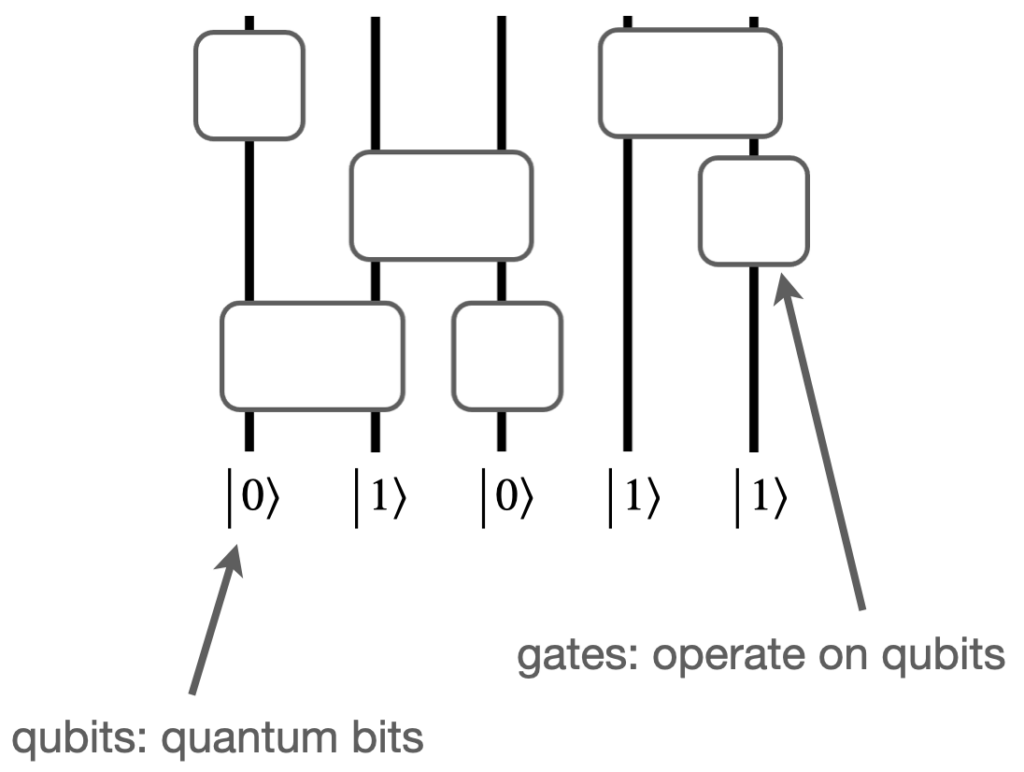
Generative artificial neural network models, such as the restricted Boltzmann machine or recurrent neural networks, are powerful candidates to encode probability distributions and efficiently generate data samples. With this power, generative neural networks can be used as a wavefunction ansatz to represent states of quantum many-body systems and generate measurement data in different bases. The networks can be trained to reconstruct quantum states from experimental measurements, to variationally search for the ground state of a given Hamiltonian, or to simulate dynamics in perturbed quantum systems.
Spiking neural networks for quantum state representation
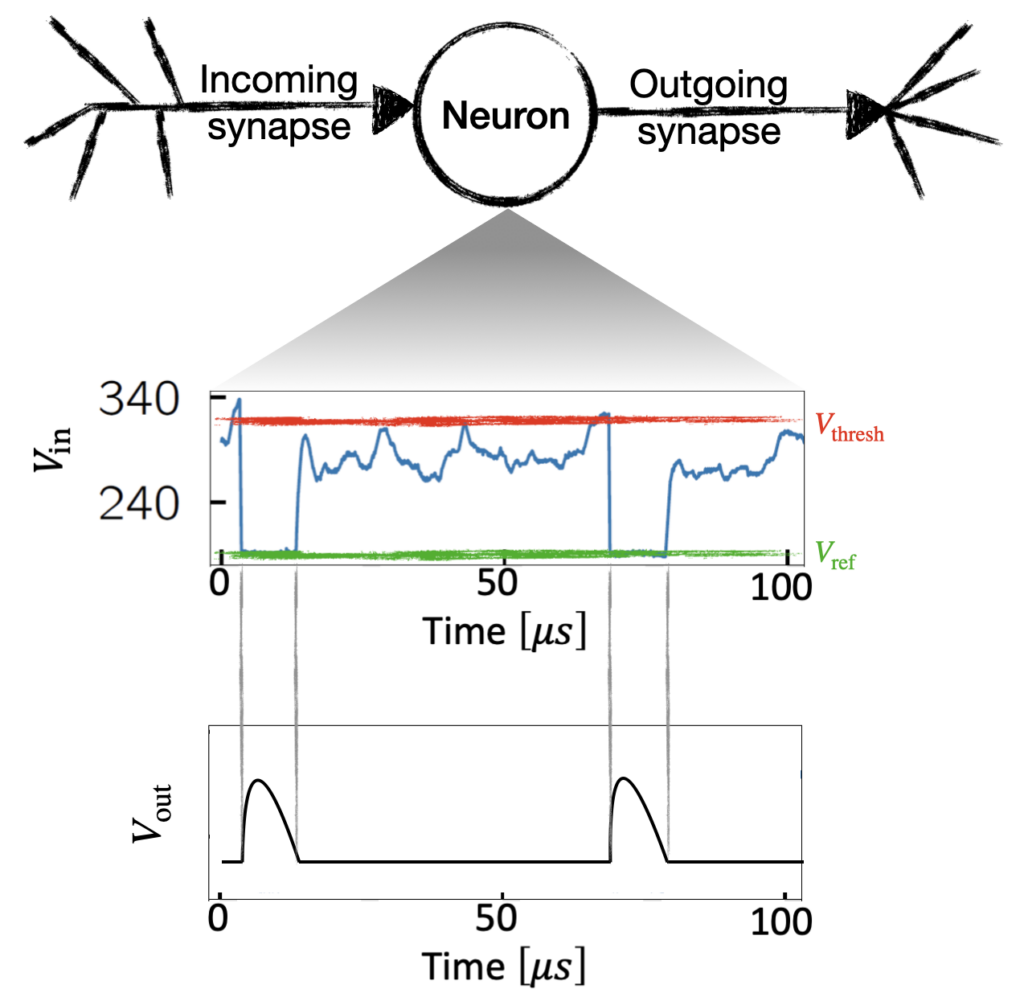
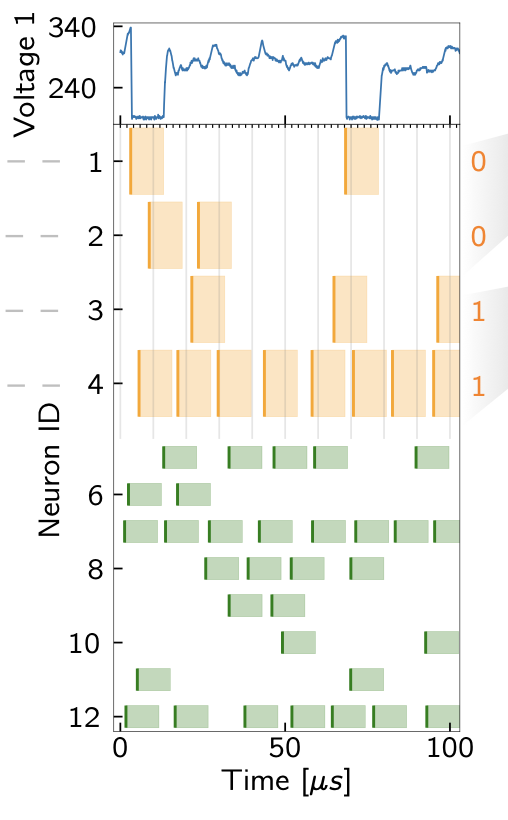
Spiking neurons mimic the behaviour of biological neurons and thus differ significantly from commonly used artificial neurons. Networks of spiking neurons commonly implement all-to-all connections, via which information is propagated in the form of time-dependent spike events. The accelerated behaviour of spiking neural networks and the possible implementation on small and energy-efficient neuromorphic hardware propose immense advances in the field of quantum state representation with generative neural networks.
Artificial and physical neural networks for quantum experiments
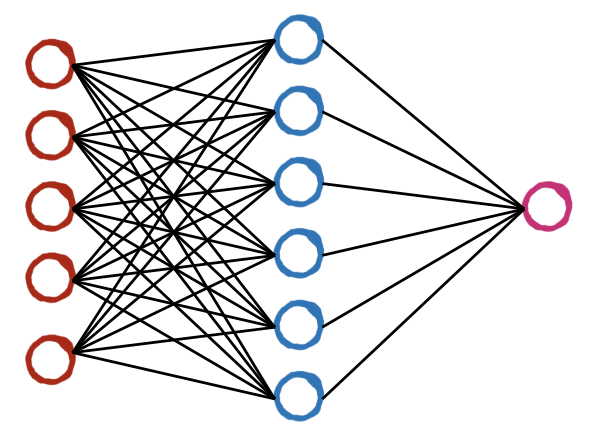

The power of artificial neural networks to automatize operational tasks can be observed in daily applications such as text prediction, speech recognition, or driving assistants. Similarly, artificial neural networks can be used to automatize processes in quantum experiments. A well-studied example are semiconducting devices which realize qubit systems in solid-state quantum dots, which can be automatically tuned into the operational regime. An implementation of the used networks in analog physical devices, such as memristor crossbar arrays, further proposes the integration of tuning operations in the experimental setup, close to the qubit system.